How Far Out Should You Forecast CLV?
Long-term vs short-term CLV models and a deeper dive into retention
Hello friends,
We’ve had a few folks ask whether their CLV model should be two years, three years, five years, or even something else. Determining the maximum time horizon for your CLV model is a tricky decision that significantly impacts the output. More important, while exploring how to set a maximum time horizon for your CLV model, we can dive deeper into measuring retention, which is the first step to understanding how to improve retention.
If you’ve just joined us, you can check out this index of previous CLV posts.
Let’s dive in!
An influential driver
First, let's recap some of the key terms we'll be discussing:
Subscriber decay curve — the best way to measure and visualize how well you retain paid subscribers; it's also the most influential driver in our CLV model.
Customer lifetime — our best guess at when a paid subscriber will cancel; said another way, it's a numerical summary of the subscriber decay curve.
Maximum customer lifetime — the point at which our forecast and model end.
Since we're discussing when a subscriber will cancel, it's worth noting that some subscribers will never cancel. I've had a paid subscription to Spotify since 2012, handing them $10 a month like clockwork. My current CLV is $1,330 (and growing!). As long as Spotify exists, I'll be a paying subscriber.
When looking at a group of paid subscribers (i.e., a "cohort"), there's a segment that will never cancel. In the streaming world, we called this the "cohort floor". When a cohort arrives at a particular percentage remaining (e.g., 20% to 30% for streaming services we worked on), the decay curve plateaus and the likelihood of anyone from that cohort canceling is virtually zero. Improving long-term retention and pushing up the “cohort floor” is crucial to increasing CLV and driving long-term value.
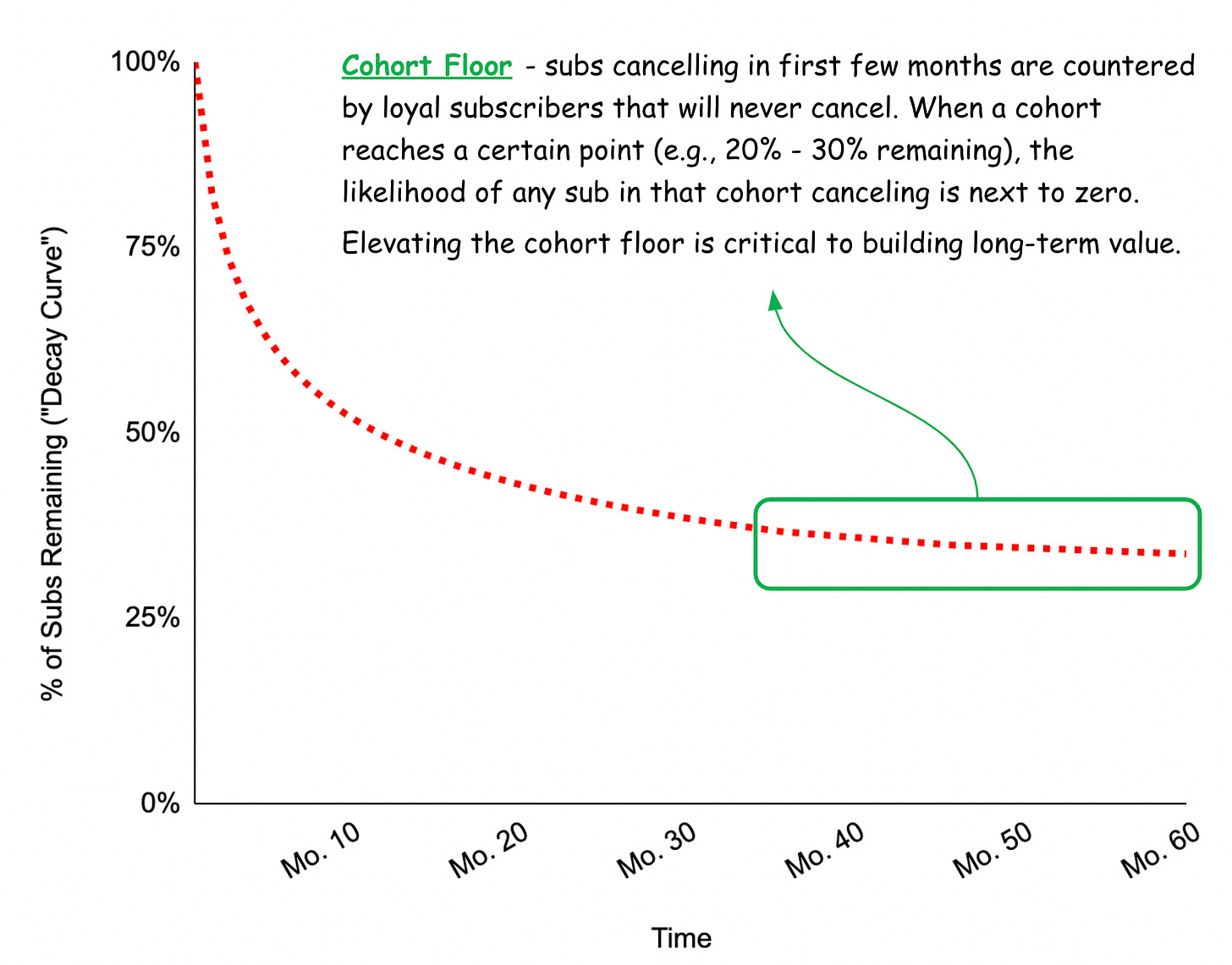
However, to model customer lifetime value, we have to set a maximum customer lifetime, the point at which our model ends, which can be a decisive assumption. In our CLV model, we’ve created tabs for a 3-year max lifetime and a 5-year max lifetime to accompany our existing 2-year max lifetime. Without changing any other assumption or driver, the table below summarizes how changing the maximum customer lifetime impacts CLV:
While there are guardrails to choosing a maximum customer lifetime, ultimately, it’s a bit subjective. It depends on where you are in your journey (e.g., just launched vs. years in), your plans (e.g., testing the waters vs. full-time and investing in growth), and the degree to which you want to be conservative or ambitious.
What’s the right max customer lifetime?
In general, the longer our CLV model extends, the riskier. We're trying to predict the future. We're far more likely to accurately predict what will happen tomorrow than five years from now. As we get further from the present moment, the cone of possibilities expands, and the model takes on more risk of being inaccurate.
Let's bring back our friend Big Dean — our (imaginary) operator of the newsletter Big Dean's Boardwalk Wings — to explore when to choose a shorter or longer max customer lifetime.
When Big Dean launches the newsletter, he’s doing it mostly as an experiment. He's unsure if this will be a long-term commitment and has a history of rotating through whimsical ideas. Also, Big Dean is fortunate to have the only restaurant near an incredibly popular beach boardwalk and pier, so most of his income comes from his restaurant and merchandise sales. The newsletter is merely a side hustle (for now). In this scenario, it may be prudent to be conservative and assume a 12- or 24-month max lifetime. Who knows if Big Dean's newsletter will even exist for that long?
But after a handful of months, Big Dean realizes he might be on to something. Subscriber growth has exploded out of the gate — people love the wing recipes and wild, behind-the-scenes stories from the restauranteur. The newsletter is helping sell even more t-shirts and drawing lines of people to the restaurant. Also, he now has a better understanding of his audience and data on how well he's retaining existing subscribers.
In this more committed and informed scenario, Big Dean is ready to take the newsletter more seriously and consider different ways to invest in growing it. At this point, Big Dean could extend his CLV model to a 24- to 36-month max lifetime.
To summarize, here’s a framework for how to choose a maximum customer lifetime:
CLV does not equal up-front cash
A CLV model is good at shooting out a single number, which can feel comforting and leave us feeling like we own our destiny. But it’s important we don’t take the CLV output as scripture. We’re trying to predict future revenue. Longer horizons not only have a wider margin of error — they require more dedication and patience.
For example, if you’re making decisions and investments based on a 5-year CLV model, you’re making a commitment to continue the business for at least five years. Given the nature of subscription models, the product must, at a minimum, maintain its value for all five years. If your product erodes in value or you stop shipping it for a few months, that may have a material impact on the entire decay curve.
We also need the patience to collect the predicted earnings over 60 months. Don’t fool yourself into thinking the entire CLV amount shows up in your bank account when a new sub signs up.
The shape of the curve is what matters
Everything we’ve covered is essential for understanding the basics of a CLV model. But none of it matters nearly as much as keeping an eye on your retention rates and decay curve, and having a sense of what’s driving any fluctuations.
To drive this point home, let’s look at two decay curves with the same customer lifetime but different shapes. Our green curve has better early-life retention — lower cancel rates early on but a lower “cohort floor” in the outer months. The red curve is the opposite: higher cancel rates early on, but the curve plateaus earlier (i.e., higher cohort floor).
One isn’t necessarily better than the other — they both generate the same amount of money after 2 years (i.e., the same 2-year CLV). The green curve generates more cash early on, but the red curve has a better shot at driving more revenue over time. What’s more important is that the shape of the curve tells you where you need to focus your retention efforts.
Another helpful way to think about your decay curve is to segment it into user personas. The image below divides our decay curve into three distinct groups:
Quick-churn Charlies — subs with less desire or need for your product that usually cancel in the first few months after subscribing.
Long-tenured Taylors — subs that absolutely love your product and will never cancel for as long as it’s available.
Middle-of-the-road Marias — subs that split the two groups above and resemble your average subscriber; sometimes, these subs use your product for a specific feature or element of value and cancel after they no longer need it.
If your decay curve is bending toward the red curve from above (i.e., better later-life retention), consider how to keep your quick-churn Charlies. Focusing on driving a higher frequency of usage is often a good goal for improving early-life retention. Tactically, you could improve how you onboard new users, helping them understand your product and why they need it.
If your decay curve is bending more toward the green curve, you may want to shift your retention efforts toward middle-of-the-road Marias. It’s usually best to focus on diversity and depth of usage to improve longer-term retention. Guide Marias further into the part of the product they value the most, or try introducing them to other features or aspects of your offering.
In the streaming world, to improve early-life retention, we would try to make it as easy as possible to find the hit show most people were signing up to watch. Then we would gradually encourage them to watch other new shows to drive a higher frequency of usage. Over time, we would encourage them to watch on more than one device, and eventually, guide them toward deep-catalog shows (e.g., Game of Thrones or Naruto) to deepen their engagement.
While certain tactics can focus on improving specific points in the decay curve, we usually see an improvement in early-life retention ripple through the entire curve — this is what we mean by "elevating the decay curve". Pushing the whole curve upward can drive serious value, especially as subscribers grow. Below is an example showing a 10% improvement in the entire decay curve. Check out how the gap between the dotted and solid green lines expands over time (i.e., the improvement in CLV compounds).
That feels like a good place to cut me off for now!
We now have a framework for choosing the maximum customer lifetime in your CLV model, but more importantly, we have a deeper understanding of what truly matters: the shape of the decay curve. We also walked through a few surface-level segmentation exercises to help us identify what’s moving our decay curve and how we may be able to improve retention.
What part of the post stood out to you or sparked ideas? What questions remain? Tired of me talking about decay curves? Let us know what you think in the comments.
Thank you for reading,
Reid
One of my issues is I have no idea what causes a spike or a plateau in subscriptions. Without having more data points and KPIs to inform me, it's difficult for me to tweak what I'm actually doing. What is the use of projecting forward if my conversion optimization is suboptimal and I don't actually know what I'm doing wrong? I need features in the platform that give me the appropriate feedback. Otherwise I might fail out of sheer ignorance and a lack of data.
That's not to say that CLV isn't fascinating or that various characteristics of this are not truly useful. I'm just very puzzled by the volatility of growth, making me assume these are not factors in my control.
Helpful as always! I'm learning a lot and it's making the psychological reality of becoming an independent business person easier for me!